Making Sense of Randomness
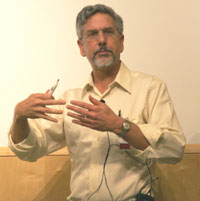
Dr Kenneth Brown, Department
Chair of Mathematics at Cornell
University.
Try predicting the weather, an election result, a game of baseball or the order of a shuffled deck of playing cards - and you might end up scratching your head. If you are ever in a casino, try predicting the outcome of the bets you are placing.
To most of us trying to predict random events might seem like an art form where hindsight is the best gift available - a gift unfortunately most of us don‘t have. Usually, we can‘t read the tealeaves, predict the future or know the outcome of the national lottery. Unless of course you are part soothsayer, part Nostradamus and have a "sixth sense".
But there is hope. Thanks to a Russian Mathematician, Andrei Andreyavich Markov (b 14 June 1856 in Ryazan, Russia; d: 1933 in Petrograd), we have a mathematical tool called "Markov Chains" which give us some help in making order out of the seemingly random. Instead of remarking "its all greek to me" we have Markov Chains to help us - at least in part.
Markov Chains were the subject of a recent lecture at WCMC-Q by visiting Department Chair of Mathematics at Cornell University, Dr Kenneth Brown. To an assembled cast of interested students and faculty, Dr Brown defined Markov Chains, detailed the fundamental theorem and areas of application.
Certain systems, such as population movement and genetics, change through a finite number of states over time and behave randomly according to certain probabilities. If the probability that the system will be in a particular state during a given time period depends only on its state during the previous time period, then the system is called a "Markov Chain".
Markov Chains or analysis looks at a sequence of events and analyzes the tendency of one event to be followed by another. They are useful processes for examining dependent random events, those events whose likelihood depends on what happened last, such as population change and movement.
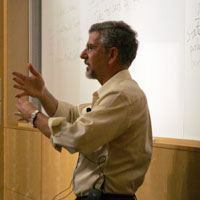
Dr Brown unravels the details
of Markov Chains.
Many random events are affected by what happened before - such as the weather or shuffling a pack of cards. Modern applications in computer programming use Markov Chains as an integral part of their functionality and marketing gurus the world over may apply them to explore how their customers and markets may evolve - powerful tools for anticipating, forecasting and experimenting with market changes.
So next time the pollsters predict an election result from random sampling techniques or you wonder how professional marketers apply their forecasting - then there‘s a probability that a Markov Chain is being applied somewhere in the process.
As Dr Brown concluded in his lecture, if you want to know more about Markov Chains or their derivative, the exotic sounding "Markov Chains Monte Carlo" browse the web search engines. Watch the number of references to Markov Chains appear, not all random, nor somewhere in the South of France!